A Comparative Analysis on Medical Article Classification Using Text Mining & Machine Learning Algorithms
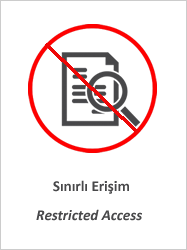
View/ Open
Access
info:eu-repo/semantics/closedAccessDate
2021Author
Kolukisa, BurakDedeturk, Bilge Kagan
Dedeturk, Beyhan Adanur
Gulşen, Abdulkadir
Bakal, Gokhan
Metadata
Show full item recordAbstract
The document classification task is one of the widely studied research fields on multiple domains. The core motivation of the classification task is that the manual classification efforts are impractical due to the exponentially growing document volumes. Thus, we densely need to exploit automated computational approaches, such as machine learning models along with data & text mining techniques. In this study, we concentrated on the classification of medical articles specifically on common cancer types, due to the significance of the field and the decent number of available documents of interest. We deliberately targeted MEDLINE articles about common cancer types because most cancer types share a similar literature composition. Therefore, this situation makes the classification effort relatively more complicated. To this end, we built multiple machine learning models, including both traditional and deep learning architectures. We achieved the best performance (R¿82% F score) by the LSTM model. Overall, our results demonstrate a strong effect of exploiting both text mining and machine learning methods to distinguish medical articles on common cancer types.